How to grow revenue
There are many balls to juggle in running a business, but lack of money is the BIGGEST. In this time of increasing levels of insolvency, typically from significant drops in sales and changing market conditions, many are getting their revenue strategy wrong and struggling to survive.
Assumptions are made that discount pricing strategies increase revenue, sales volume, utilise capacity shortfalls and make sufficient profit. Or, that the advertising (traditional, digital or otherwise) will ultimately produce revenue beyond the media and creative spend. Or, perhaps, the investment in the cold-calling sales team will generate more revenue than it irritates non-customers. Revenue generation tactics such as these may be adopted as the default status-quo strategies, more so than based on strategic merit. Yet, to ASS-U-ME they are working is potentially dangerous.
In recent years, there has been an evolution in thinking as to optimal revenue strategy. Two priority areas have emerged as mission critical to revenue growth focused businesses.
1. Growth Hacking
Evolving from traditional marketing and brand building models, often with a long fuse and unpredictable ‘bang,’ to growth hacking strategy building on data, agility (continuous learning and iteration), technology and generating revenue growth (discussed in ezine Square Holes 18 and on squareholes.com/blog here).
2. Revenue Management
The application of disciplined analytics that predict consumer behaviour at the micro-market level and optimise product availability and price to maximise revenue growth.
Rather than making assumptions as to optimal pricing, market segments and growth opportunities, revenue management applies a greater level of data gathering and strategic analysis to ensure the right products are sold to the right customers at the right time and with the right bundling. Through combining data analytics and customer insight, optimal strategies are able to be defined aligning product, price, placement and availability with each customer segment.
Revenue management has its origins back in the 1980’s to support sectors including airlines and hotels in selling capacity surplus, with early bird and discount fares, differentiated pricing models and volume rates. This followed with sectors from media to motoring increasing revenue management sophistication in the 1990’s through market segmentation and greater levels of strategic targeting to offer differentiated pricing and products to maximise revenue. Even with early skepticism, since the 2000’s many sectors have widely embraced revenue management principles, with many case studies of businesses going from struggling to survive to thriving through fundamental strategic shifts.
There is increasing acceptance that revenue management can and should be applied across broad categories from products to professional services. Particularly in this time of higher potential for underutilisation of capacity and the ever uncertain and changing market and consumer environment.
Below is a summary of 5 key steps to optimising revenue management.
1. Data
Revenue management starts with processes to collect, store and allow robust analysis across diverse relevant datasets. This may include sales data (e.g. customer data—profile, product, price, quantity etc), market data (e.g. economic conditions, weather, category data, other key measures etc), consumer data (e.g. market research data on shopping behaviours, attitudes, needs, product preferences, pricing, competitors etc), population data (e.g. Bureau of Statistics Census and other population data), media data (e.g. spend, digital metrics and paid media schedule etc), financial / operation data (e.g. P&L, inventory, capacity etc) and other relevant data.
Critical in this is reliability of data (i.e. the ability to match the reality), access (i.e. the ability to easily export the data), timeliness (i.e. the ability to analyse the data in a timely manner) and ability to analyse in an integrated manner (i.e. tracking patterns over time from one dataset with others to identify correlations, linkages from one dataset to the next—e.g. linking demographic and/or membership data from surveys to Australian Bureau of Statistics and membership data, overlaying mapping data from various datasets).
New data collection, storage and analysis tools are ever emerging and evolving to allow this with greater ease.
2. Segmentation
The second priority of revenue management is using the collected data to segment the market into unique clusters—evidence based groups of current and potential customers who are statistically identical within each segment and statistically distinguished between segments.
Statistical analysis methodology cluster analysis is widely used to define segments based on behavioural, demographic, attitudinal, spend and other data from surveys and, when possible, integrated with other data to provide deeper and wider insight.
This provides revenue management priorities including the overall market size (i.e. market value, number of customers and market share of competitors); relative potential of market segments (i.e. which segments are the largest by current and potential value—size, spend, frequency of spend and other variables); unique needs in terms of product, pricing etc; and strategic priorities (i.e. media consumption / impact, distribution etc).
What are the consistent truths irrespective of market segment, and may be a valuable broader market message, and how can valuable niche segments be optimally targeted and revenue maximised?
Typically businesses humanise the market segments (i.e. bring them to creative life) via visual and data design, combining the quantitative data from various sources, defining segments via cluster analysis and then conducting qualitative research including focus groups, affinity and in-home discussions, ethnography, semiotics and other analysis of cultural and consumer patterns and trends to provide greater strategic empathy and a platform for revenue management.
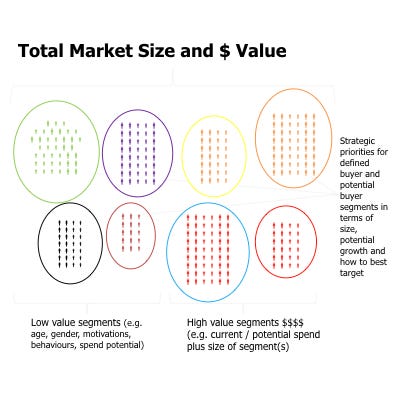
3. Forecasting
Building on the understanding of the broader market and unique segments, forecasting seeks to model the price elasticity.
This likely includes measuring the willingness to pay for the product, bundles and distinctive products for the broader market and unique segments. For example, one segment may be more aware of the value of a higher quality product, and with this display a higher willingness to pay than other segments, who may be more price sensitive.
Analysis may be of historic sales and other data as to when prices and other factors moved, the corresponding impact on sales. In addition to this, market research provides simple and more complex price and revenue modelling methodologies and scenario planning approaches from surveys asking for reasonable and maximum price willingness to pay and mapping the likely demand, to more complex approaches such as choice modelling, brand-price trade-off modelling and other models.
Qualitative research such as focus groups and in-depth interviews can be valuable in exploring new product offerings, that can then be measured in quantitative survey research, which can then be cross-referenced with sales and other market data.
4. Optimisation
With an understanding of universal market truths, segment differentiation priorities and forecasting as to likely market and segment revenue response to pricing changes, is a need to set strategic priorities as to what the business needs to do to develop an optimised revenue management strategy.
Key considerations need to be taken into account—optimal pricing, total sales potential and internal budgets, cost of goods sold, margins and other key financial metrics and customer lifetime value. It is therefore critical to understand which levers can be adjusted to have the optimal revenue impact.
One valuable approach to prioritising strategies is taking the data collected and understanding of the unique market segments, and conducting regression analysis to define strong correlations between movements in one variable (e.g. perceived performance in quality or availability; and/or actual sales or media spend) on overall revenue.
This removes potentially risky analysis in asking potential customers ‘what would encourage you to buy more?’ to indirect analysis of the key ‘hidden’ and even seemingly irrational drivers as to why some customers buy more than others, provide insight into sales fluctuations and identify strategic priorities.
An extension of this is incorporating econometrics to define revenue management models, which can provide a mission critical strategic and scenario planning framework.
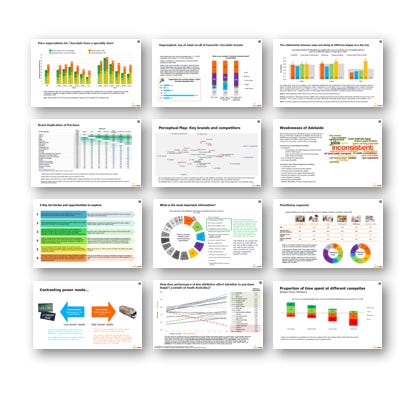
5. Agility
Most importantly, revenue management is an ever learning and iterating process. Action, evaluate, learn, iterate and repeat, ever seeking to maximise revenue and reduce underutilisation of capacity. This may infer the tweaking and customising of products to best meet prior market segment needs, or dumping products no longer with sufficient market demand.
Constant reassessment of the promotion and distribution strategies, and emerging market opportunities domestically and export.
The commitment to being data driven in the pursuit of the optimal revenue model is not complex. It just requires a commitment to a systematic and integrated process to ensure the right products are sold to the right customers at the right time and with the right bundling.
Rather than assuming that the market fits default revenue strategies, theories and likely outdated category models, revenue management principles offer much opportunity.
What do you think? We’d love to hear any thoughts. Please share and/or leave a comment. If you would like to discuss how Square Holes may be able to help with the above, please get in touch.
🙂