How to do product research (not one of the 95% new products that FAIL)
It is believed that only one in twenty products launched into market succeed. And, while failure is said to be the new success, wouldn’t it be cheaper, less risky and better for all to try to plan for success?
The 95% failure rate comes from research by Harvard Business School Professor Christensen published in 2011. While seven years ago, likely the fail rate is not falling, even growing as the ease to and quickly build, pack and send a physical or digital products is better than ever for anyone with an idea. Rapid prototyping, agile scrumming, co-creation, brainstorming sprints and workshops and fantastic innovations and they offer a great deal. Yet they do risk teams getting lost in category confirmation bias, group think and the organisation norm, rather than truly understanding the market potential of their new product idea.
With this, the question is whether such high failure rates is simply a sunk cost or a reality of launching products, or can it be reduced? Perhaps we should ask the potential customers. Is there an actual product-market fit?
What are the optimal product, price and other elements optimal?
This all sounds a bit boring. Particularly for those shoot-them-up-cowboy emulating entrepreneurs, preferring to ride into town ‘guns’ blazing with ‘the best darn product this side of Texas’ never fearing their product is more fizz than bang.
Research is of value in the primary customer understanding that can be critical in understanding market gaps, opportunities or category / product wrong(s) that need to be made right. It can also be invaluable in testing products qualitatively and quantitatively to identify the likely demand, gaps and opportunities for refinement, as well as which product combination (i.e. elements, pricing, size etc) is likely optimal across the market to maximise revenue and profitability amongst key segments.
Will people buy the product, and what is the price sweet spot?
There are three broad ways research can help product testing and refinement.
Monadic Testing
Basically testing ‘one’ concept at a time and seeking feedback. Be this in qualitative research (e.g. focus groups, in-depth interview etc) or quantitative research (e.g. surveys), monadic testing essentially refers to more deeply exploring a single product concept against key factors (e.g. appeal, likely usage, price point, likes, dislikes, ratings in key products attributes such as size, capacity etc). Monadic testing can incorporate testing such as heat mapping of key areas of appeal, eye catching etc; recording video / audio response and emotional assessment of the concept.
This can be then followed by a comparison of alternatives in situations where there are multiple concepts developed. Such research can be valuable in deep exploration of a concept (and suite of concepts in isolation and then comparatively), to allow for assessment of likely product — market fit, optimal path to market, required refinements, and key strategic considerations — e.g. pricing, critical attributes and other factors.
A/B Testing
Common in testing of digital concepts such as websites and email campaigns, A/B testing is valuable when testing the appeal of one concept over another. In a digital setting, A/B testing can record key metrics such as click through and other calls to action. In a qualitative (e.g. focus groups) or quantitative (e.g. surveys) research setting, A/B testing can be an efficient way of quickly testing factors such as the relative appeal of two concepts. Surveys with large representative samples, can allow for the appeal of alternative concepts to measured over the total market and market segments based on demographics, buyer profile and other total market segmentation.
Predicative Testing
Research can also play a role in modelling the optimal product to fit the market and predict factors such as optimal pricing, revenue and segment differentiation. Statistical approaches such as Choice Based Conjoint, aka CBS or discrete choice conjoint analysis, to ask users to choose between a limited set of alternatives against fictional products or services with a number of stated characteristics (e.g. size, weight, performance, price etc), and asking respondents choose between alternatives. The question is then repeated multiple times with varying random combinations of characteristics. This allows for the analysis of factors such as what is important and what prospective customers are willing to trade off. This is useful when often consumers may not be consciously aware and/or able to articulate preferences without such an approach.
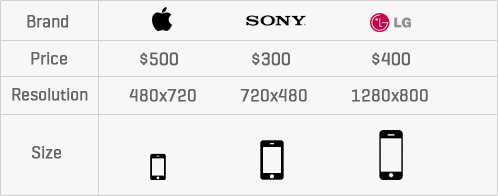
Analysis of the outcomes of choice-based conjoint allows for the customer utility preferences to be defined via advanced statistical analysis such as multinominal logistic regression, maximum likelihood estimation, Bayesian techniques and other advanced algorithms. Analysis allows for insights such as the relative importance percentages, such as the the optimal price point, size and the relative importance between factors such as brand, price, resolution and size. For example, does Apple, or the new product’s brand, represent a particularly strong utility and able to demand a price premium?
Conjoint analysis can also allow for measurement of preferences, predictive market share of various product concepts, optimal pricing, price elasticity, revenue / profit maximisation, customer segmentation, optimal positioning and willingness to pay more/ less depending on the brand.
Such analysis allows for the necessary analysis and planning to NOT fail and to be in the 5% of products that succeed, rather than blindly accepting failure as the new success.
As failing sucks.